Abstract's details
Deep learning for accurate SSH reconstruction from altimetry and SST observations
CoAuthors
Event: 2022 Ocean Surface Topography Science Team Meeting
Session: Science III: Mesoscale and sub-mesoscale oceanography
Presentation type: Type Poster
Contribution: PDF file
Abstract:
Two-dimensional sea surface height (SSH) reconstructions from satellite altimetry are widely used in studies of upper ocean dynamics. Nonetheless, accurate SSH reconstruction is challenging since observations are only made along widely spaced one-dimensional tracks, leaving large observational gaps. The widely used SSALTO/DUACS product implements optimal interpolation (OI) to reconstruct the SSH field from the along-track altimetry. However, OI introduces significant errors in regions of high eddy kinetic energy, where the dynamical timescales become comparable to the satellite return times. Improvements will likely be achieved if the interpolation procedure succeeds in accounting for the non-linear multi-scale dynamics of mesoscale ocean turbulence and its interactions with the evolving mean flow.
Recent studies across many disciplines demonstrate that Deep learning (DL) neural networks can reveal complex non-linear dependencies in large datasets, and we have adapted this approach to satellite altimetry. Here, we present an improved SSH reconstruction using a DL framework that is based on a convolutional long short-term memory architecture. In addition to satellite altimetry, we also utilised sea surface temperature (SST) observations to achieve a significantly lower SSH reconstruction error than existing interpolation methods. Tested on independent altimeter observations for a region of the Gulf Stream with an energetic mesoscale eddy field, our high-resolution reconstruction (7km grid) has about a 30% improvement in root-mean-square error relative to SSALTO/DUACS. While already providing a more accurate and higher-resolution SSH product, our DL framework could be improved in many ways, including using different types of existing ocean datasets and wide-swath observations from the upcoming NASA SWOT and ODYSEA missions.
Recent studies across many disciplines demonstrate that Deep learning (DL) neural networks can reveal complex non-linear dependencies in large datasets, and we have adapted this approach to satellite altimetry. Here, we present an improved SSH reconstruction using a DL framework that is based on a convolutional long short-term memory architecture. In addition to satellite altimetry, we also utilised sea surface temperature (SST) observations to achieve a significantly lower SSH reconstruction error than existing interpolation methods. Tested on independent altimeter observations for a region of the Gulf Stream with an energetic mesoscale eddy field, our high-resolution reconstruction (7km grid) has about a 30% improvement in root-mean-square error relative to SSALTO/DUACS. While already providing a more accurate and higher-resolution SSH product, our DL framework could be improved in many ways, including using different types of existing ocean datasets and wide-swath observations from the upcoming NASA SWOT and ODYSEA missions.
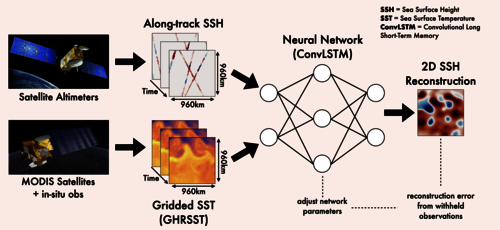