Abstract's details
Attenuating the ocean chaotic variability in altimetric observations: from band-pass filtering to machine learning
CoAuthors
Event: 2019 Ocean Surface Topography Science Team Meeting
Session: Science II: Large Scale Ocean Circulation Variability and Change
Presentation type: Type Oral
Contribution: PDF file
Abstract:
The OCCIPUT large-ensemble global ocean simulation (https://meom-group.github.io/projects/occiput/) has been used to partition the multi-scale ocean variability into deterministic (forced by the atmosphere) and chaotic (ocean-generated, random) signals. Further studies have shown that these chaotic signals can be large enough in many regions to mask deterministic signals, and hinder the local detection and attribution of climate change in the ocean, such as regional trends in ocean heat content and sea level.
It is thus tempting to try and attenuate the signature of chaotic variability in observational datasets, in order to unveil its atmospherically-forced component, and help identifying the external drivers of the observed variability.
We first developed a band-pass spatial filter, based on the analysis of the OCCIPUT simulation outputs, which proved able to recover from a single member evolution a significant amount of the deterministic variability (0.9 correlation in global average). Applying this filter on real altimetric observations enhances the North Atlantic sea-level variability explained by the NAO by 10% to 60% locally.
An alternative to this filter, based on the U-Net convolutional neural network trained on the OCCIPUT data, showed slightly better skills on the same task, with additional benefits. The attached figure illustrates the observed (top) and U-Net-processed (bottom) SLA fields on Sept. 10th 2016, showing respectively the entangled deterministic/chaotic SLA state in the real ocean on that week, and its estimated deterministic part.
The spectral characteristics of both algorithms (filter and U-Net) is investigated in various regions; we will discuss these results, as well the possible uses of these “denoised” observational data.
It is thus tempting to try and attenuate the signature of chaotic variability in observational datasets, in order to unveil its atmospherically-forced component, and help identifying the external drivers of the observed variability.
We first developed a band-pass spatial filter, based on the analysis of the OCCIPUT simulation outputs, which proved able to recover from a single member evolution a significant amount of the deterministic variability (0.9 correlation in global average). Applying this filter on real altimetric observations enhances the North Atlantic sea-level variability explained by the NAO by 10% to 60% locally.
An alternative to this filter, based on the U-Net convolutional neural network trained on the OCCIPUT data, showed slightly better skills on the same task, with additional benefits. The attached figure illustrates the observed (top) and U-Net-processed (bottom) SLA fields on Sept. 10th 2016, showing respectively the entangled deterministic/chaotic SLA state in the real ocean on that week, and its estimated deterministic part.
The spectral characteristics of both algorithms (filter and U-Net) is investigated in various regions; we will discuss these results, as well the possible uses of these “denoised” observational data.
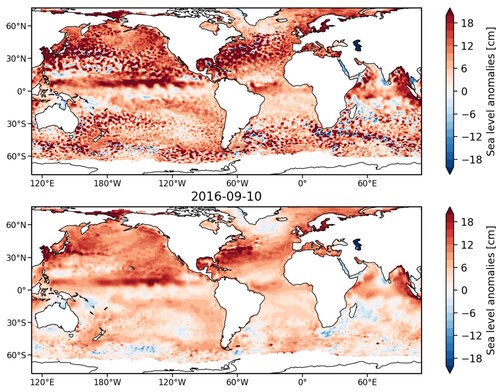