Abstract's details
Variational Deep Learning-based interpolations of along-track Nadir and wide-swath SWOT altimetry observations
CoAuthors
Event: 2020 Ocean Surface Topography Science Team Meeting (virtual)
Session: Application development for Operations
Presentation type: Type Forum only
Contribution: PDF file
Abstract:
Over the last years, a very active field of research aims at exploring new data-driven and learning-based methodologies to propose computationally efficient strategies able to benefit from the large amount of observational remote sensing and numerical simulations for the reconstruction, interpolation and prediction of high-resolution derived products of geophysical fields. In this work, we investigate how they might help to solve for the oversmoothing of the state-of-the-art optimal interpolation (OI) techniques in the reconstruction of sea surface height (SSH) spatio-temporal fields. We focus on a small 10° time 10° GULFSTREAM region mainly driven by energetic mesoscale dynamics. Based on Observation System Simulation Experiments (OSSE), we will use the the NATL60 high resolution deterministic ocean simulation of the North Atlantic to generate two types of pseudo altimetric observational dataset: along-track nadir data for the current capabilities of the observation system and wide-swath SWOT data in the context of the upcoming SWOT mission.
We demonstrate how neural networks drawing from a variational formulation of the space-time interpolation problem consists in a significant improvement of the current capabilities of the conventional methods by catching up the small scales ranging up to ~60km. A clear gain is also demonstrated when assimilating jointly wide-swath SWOT and (agreggated) along-track nadir observations.
Along this line, the application of this variational neural network framework to Observation System Experiments (OSE) on real along-track altimetric datasets is considered in the recent CLS OSTST 2020 proposal entitled "DUACS HR: Multimission merging for High Resolution Altimetry".
We demonstrate how neural networks drawing from a variational formulation of the space-time interpolation problem consists in a significant improvement of the current capabilities of the conventional methods by catching up the small scales ranging up to ~60km. A clear gain is also demonstrated when assimilating jointly wide-swath SWOT and (agreggated) along-track nadir observations.
Along this line, the application of this variational neural network framework to Observation System Experiments (OSE) on real along-track altimetric datasets is considered in the recent CLS OSTST 2020 proposal entitled "DUACS HR: Multimission merging for High Resolution Altimetry".
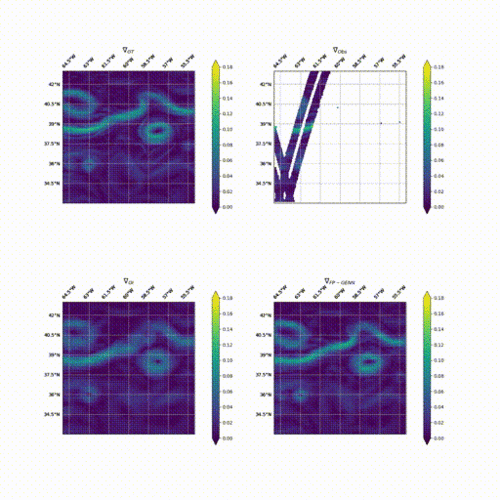